Bernardo Magnini
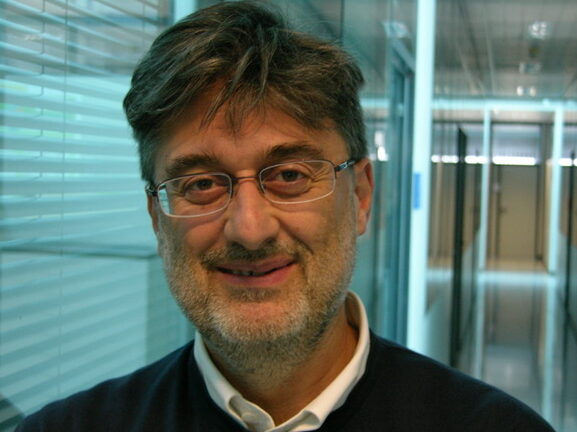
Bernardo Magnini is senior researcher at FBK (Trento, Italy), where he is responsible of the NLP research group. His interests are in the field of Computational Linguistics, particularly lexical semantics and lexical resources, question answering, semantic inferences, and conversational agents.
He has been local organizer of ACL 2019 in Florence, and General Chair of ACL 2022, the 60th Annual Meeting of the Association for Computational Linguistics, in Dublin. He has been President of the Italian Association for Computational Linguistics (AILC) from 2015 to 2022.
Giovanni Bonetta
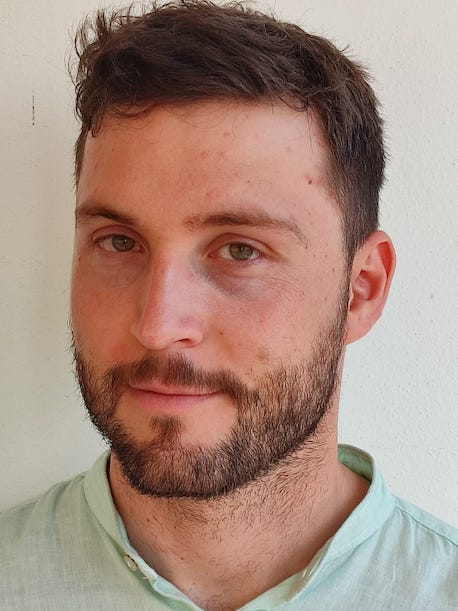
Giovanni Bonetta is currently a researcher in the NLP Research Group at the Bruno Kessler Foundation (FBK), where he focuses on developing and integrating Large Language models and Visual Language models within the Italian context, exploring their applications in embodied systems, and benchmarking their capabilities. Previously, he held a postdoctoral position at the University of Turin’s Computer Science department, where he also achieved his Ph.D. in 2022. During this time, he worked on sparsity in deep generative models and applied Reinforcement Learning to Combinatorial Optimization. His Ph.D. was industrial, completed in collaboration with Nuance Communications, where he specialized in data-to-text generation and chatbot development, contributing to the Nuance Agent Coach platform.
You Are what You Eat: Processing Data for Training and Evaluating LLMs
Data is becoming one of the most critical ingredients for developing Large Language Models (LLMs), as the models’ behavior is largely affected by both the amount and the quality of training data. In addition, high quality data drives LLMs evaluation, with relevant implications not only for research but also for the application market. Finally, if we focus on data about different languages, we can not ignore that the availability of such data is highly unbalanced toward very few languages. The tutorial addresses key aspects related to the use of textual data for LLMs, and, with less emphasis, of multimodal data. Specifically, we describe a pipeline for data preparation, covering, among other steps, collection, cleaning, deduplication, and filtering. We highlight some of the most used data repositories for training and finetuning LLMs, including multilingual data. We then survey the legal issues related to using data, including potential violation of regulations about copyright, about the privacy of personal information and about the potential generation of both offensive content and misinformation. When it comes to processing data for benchmarking LLMs, the tutorial shows recent works in this area, with particular emphasis on benchmarks for Italian, including those derived from English translations and those originally created in Italian.